A new study by researchers from Carnegie Mellon University, NVIDIA, Ariel University, and Microsoft has proposed a two-stage approach called SPRING (Study-Prompt-Reasoning-Inference-Navigate-Guide). The prompting framework outperforms the traditional reinforcement learning approach for training intelligent agents. The study primarily focused on using Large Language Models (LLMs) for understanding and reasoning with human knowledge in the context of games.
LLMs are long-form language models with attention designed to understand and process large amounts of text data. Previously, the traditional method for training intelligent agents was Reinforcement Learning. However, it requires a lot of data and could be computationally expensive. The researchers found that their SPRING framework outperformed the Reinforcement Learning approach of training LLMs.
Also Read: NVIDIA Towards Being The First Trillion Dollar AI Chip Company
What is SPRING?
SPRING is a prompting framework designed to enable a chain of thoughtful planning and reasoning for LLMs. It generates prompts that guide the models toward a particular thinking or goal. The framework uses a ” strategic prompting technique,” which involves predicting the next logical step in a conversation based on the previous context.
Also Read: Prompt Engineering: Rising Lucrative Career Path AI Chatbots Age
How Does SPRING Work?
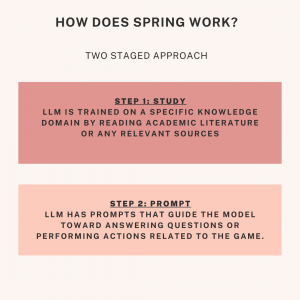
The SPRING prompting framework is divided into two stages:
Stage 1: Study
The first stage involves studying an academic paper to obtain knowledge to the game. In this stage, the LLMs are trained in a specific knowledge domain by reading academic literature or relevant sources to acquire essential information.
Stage 2: Prompt
In the second stage, the LLMs have prompts that guide the model toward answering questions or performing actions to the game. The prompts generated by SPRING are dynamic and depend on the context of the conversation rather than relying on a pre-determined set of prompts. If the user asks a question, the framework generates a prompt that leads the discussion toward answering that question.
Learn More: An Introduction to Large Language Models (LLMs)
Why Does SPRING Outperforms Reinforcement Learning?
The SPRING framework generates prompts based on the conversation’s context, allowing for a more natural flow of dialogue. In contrast, Reinforcement Learning requires a lot of data and can be computationally expensive. Furthermore, Reinforcement Learning relies heavily on trial and error. At the same time, SPRING guides the LLMs toward a particular line of thinking or goal.
Learn More: Understanding Reinforcement Learning from Human Feedback
Our Say
The SPRING prompting framework has shown promising results in training LLMs. The dynamic prompts generated by the model enable a more natural flow of conversation. Thus, making it easier to train intelligent agents without requiring massive amounts of data. As AI technology evolves, frameworks like SPRING will become essential in developing more advanced and efficient language models and will keep outperforming traditional approaches.
By Analytics Vidhya, May 30, 2023.