Introduction
DreamFusion, the revolutionary AI-driven technology capable of converting text into 3D visuals using 2D diffusion techniques, has recently been honored with the prestigious Best Paper Award at ICLR-2023. This accolade is a testament to the groundbreaking research and innovation behind DreamFusion, as well as its potential to transform numerous industries.
In this blog post, we’ll take a closer look at the significance of this achievement, the research that led to DreamFusion’s success, and the potential implications for the future of text-to-3D visualization.
DreamFusion: An Overview
At its core, DreamFusion is a revolutionary AI technique that converts text-based input into detailed 3D models. It achieves this by utilizing a cutting-edge machine-learning algorithm known as 2D diffusion. By training the AI system on vast datasets of images and associated textual descriptions, DreamFusion is able to generate intricate and realistic 3D representations based on user-provided text.
The Power of 2D Diffusion
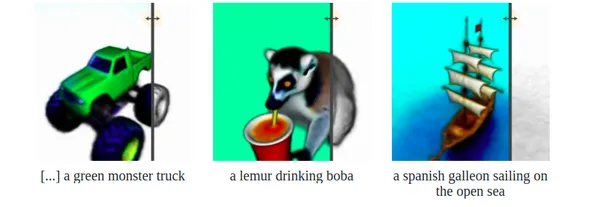
2D diffusion is a generative modeling technique that has been applied in various AI applications, such as image synthesis and inpainting. It involves a stochastic process that gradually adds or removes detail from an image in a controlled manner. By learning the intricate relationships between pixels, 2D diffusion models are capable of generating high-quality images with remarkable levels of detail.
In the context of DreamFusion, 2D diffusion plays a critical role in translating textual input into 3D models. By iteratively refining a 2D representation of the model, the algorithm gradually adds depth and complexity to create the final 3D object. This allows DreamFusion to generate visually stunning and accurate 3D models based solely on text descriptions.
The DreamFusion Process: Step by Step
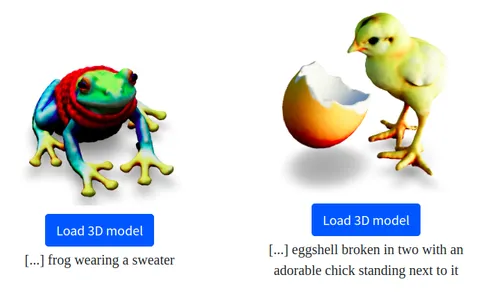
- Text Input: The user provides a textual description of the desired 3D model. This description should be concise yet detailed, providing sufficient information for the AI to generate an accurate representation.
- Initial 2D Image Generation: DreamFusion’s AI algorithm leverages its training on vast image and text datasets to generate an initial 2D image that visually represents the user’s description.
- Diffusion Process: The 2D image undergoes a series of iterations, with the diffusion algorithm gradually refining and adding detail to the image. This iterative process is controlled by a series of learned parameters that guide the AI toward generating a realistic representation of the object.
- 3D Model Generation: Once the 2D image has reached an adequate level of detail, DreamFusion employs a depth estimation technique to convert the 2D representation into a 3D model. This process results in a detailed and accurate 3D model that closely matches the user’s textual description.
- Post-processing and Refinement: The user can review the generated 3D model and provide feedback or request adjustments. DreamFusion can then fine-tune the model based on the user’s input, ensuring that the final product meets their expectations.
Applications & Potential Use Cases of DreamFusion
DreamFusion’s unique ability to generate 3D models from textual descriptions opens up a world of possibilities across numerous industries.
Entertainment and Gaming:
The development of rich, immersive environments and characters is essential in the entertainment and gaming industries. DreamFusion streamlines the creation of 3D assets, allowing designers to easily transform their ideas into detailed and engaging virtual worlds. With simple textual inputs, artists can generate a wide array of 3D models, expediting the development process.
Architecture and Interior Design:
Architects and interior designers can leverage DreamFusion to bring their ideas to life, effortlessly converting written descriptions into 3D visualizations. Clients can better understand and visualize proposed designs, and designers can quickly iterate on ideas and make adjustments based on feedback. This saves time and reduces the chances of miscommunication and costly changes during construction.
Education and Training:
Educational institutions can harness DreamFusion’s capabilities to create interactive and engaging learning experiences for students. Complex concepts and ideas can be easily transformed into 3D visualizations, enhancing understanding and retention. Moreover, this technology can be used to create customized training materials for various industries, including healthcare, engineering, and aviation.
Advertising and Marketing:
DreamFusion can be used to create compelling marketing and advertising campaigns by generating lifelike 3D visuals from creative briefs. Agencies can quickly develop product mock-ups, scene visualizations, and other marketing assets to showcase their ideas to clients, ultimately resulting in more effective and persuasive campaigns.
Prototyping and Manufacturing:
DreamFusion’s ability to transform textual descriptions into 3D models can significantly streamline the prototyping process in manufacturing. Engineers can quickly create and modify designs based on specifications, reducing the time and effort required to bring new products to market. Additionally, this technology can aid in identifying potential design flaws before production, minimizing costly errors and delays.
Conclusion
DreamFusion, with its innovative approach to text-to-3D conversion using 2D diffusion techniques, is poised to transform numerous industries and revolutionize the way we visualize and create 3D content. By making it possible to generate detailed, accurate, and aesthetically pleasing 3D models from simple textual descriptions, DreamFusion is democratizing the world of 3D design and opening up new avenues for creativity and innovation.
As the technology continues to advance, we can expect even greater levels of detail, accuracy, and realism in the 3D models generated by DreamFusion. The future holds exciting possibilities for creators, professionals, and businesses alike as they harness the power of this groundbreaking tool to bring their visions to life in vivid three-dimensional detail.
Read the full article here