The NeurIPS 2024 Best Paper Awards were announced, spotlighting exceptional contributions to the field of Machine Learning. This year, 15,671 papers were submitted, of which 4,037 were accepted, representing an acceptance rate of 25.76%. These prestigious awards are the result of rigorous evaluation by specialized committees, comprising prominent researchers with diverse expertise, nominated and approved by the program, general, and DIA chairs. Upholding the integrity of the NeurIPS blind review process, these committees focused solely on scientific merit to identify the most outstanding work.
What is NeurIPS?
The Conference on Neural Information Processing Systems (NeurIPS) is one of the most prestigious and influential conferences in the field of artificial intelligence (AI) and machine learning (ML). Founded in 1987, NeurIPS has become a cornerstone event for researchers, practitioners, and thought leaders, bringing together cutting-edge developments in AI, ML, neuroscience, statistics, and computational sciences.
The Winners: Groundbreaking Research
This year, five papers—four from the main track and one from the datasets and benchmarks track—received recognition for their transformative ideas. These works introduce novel approaches to key challenges in machine learning, spanning topics like image generation, neural network training, large language models (LLMs), and dataset alignment. Here’s a detailed look at these award-winning papers:
NeurIPS 2024 Best Paper in the Main Track
Paper 1: Visual Autoregressive Modeling: Scalable Image Generation via Next-Scale Prediction
Here’s the Paper: Link
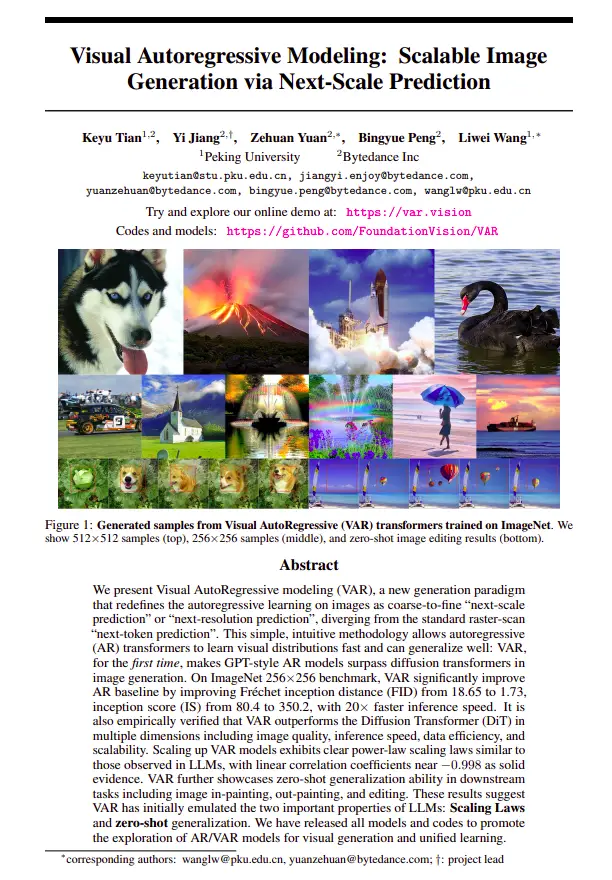
Author: Keyu Tian, Yi Jiang, Zehuan Yuan, BINGYUE PENG, Liwei Wang
This paper introduces a revolutionary visual autoregressive (VAR) model for image generation. Unlike traditional autoregressive models, which predict subsequent image patches based on an arbitrary order, the VAR model predicts the next higher resolution of the image iteratively. A key component is the innovative multiscale VQ-VAE implementation, which enhances scalability and efficiency. The VAR model surpasses current autoregressive methods in speed and delivers competitive results against diffusion-based models. The research’s compelling insights, supported by experimental validations and scaling laws, mark a significant leap in image generation technology.
Paper 2: Stochastic Taylor Derivative Estimator: Efficient Amortization for Arbitrary Differential Operators
Here’s the Paper: Link
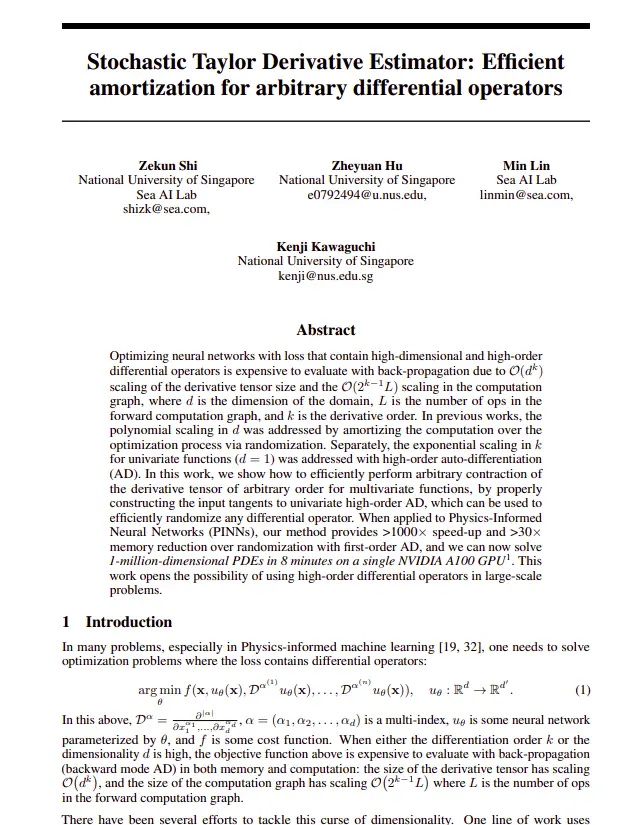
Author: Zekun Shi, Zheyuan Hu, Min Lin, Kenji Kawaguchi
Addressing the challenge of training neural networks (NN) with supervision incorporating higher-order derivatives, this paper presents the Stochastic Taylor Derivative Estimator (STDE). Traditional approaches to such tasks, particularly in physics-informed NN fitting partial differential equations (PDEs), are computationally expensive and impractical. STDE mitigates these limitations by enabling efficient amortization for large-dimensional (high ddd) and higher-order (high kkk) derivative operations simultaneously. The work paves the way for more sophisticated scientific applications and broader adoption of higher-order derivative-informed supervised learning.
NeurIPS 2024 Best Paper Runners-Up in the Main Track
Paper 3: Not All Tokens Are What You Need for Pretraining
Here’s the Paper: Link
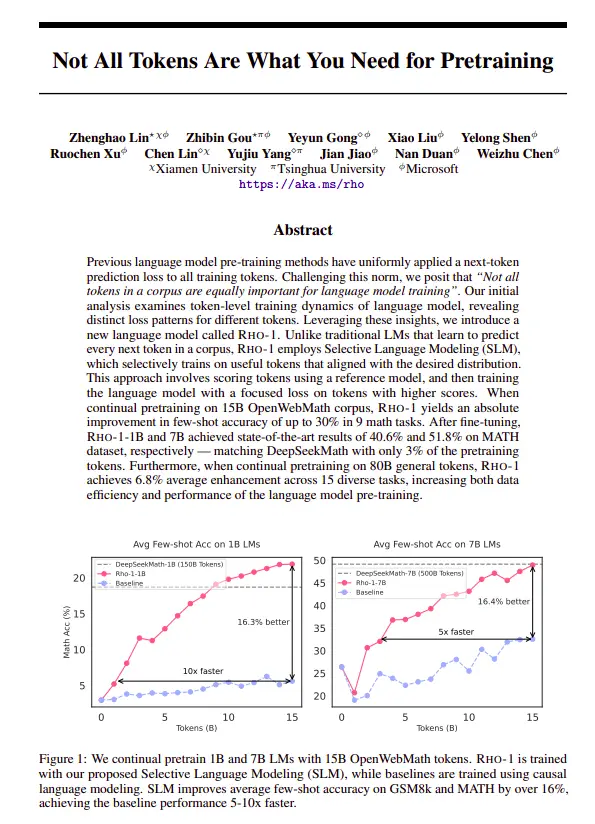
Author: Zhenghao Lin, Zhibin Gou, Yeyun Gong, Xiao Liu, yelong shen, Ruochen Xu, Chen Lin, Yujiu Yang, Jian Jiao, Nan Duan, Weizhu Chen
This paper proposes an innovative token filtering mechanism to improve the efficiency of pretraining large language models (LLMs). By leveraging a high-quality reference dataset and a reference language model, it assigns quality scores to tokens from a broader corpus. High-ranking tokens guide the final training process, enhancing alignment and dataset quality while discarding lower-quality data. This practical yet effective method ensures LLMs are trained on more refined and impactful datasets.
Paper 4: Guiding a Diffusion Model with a Bad Version of Itself
Here’s the Paper: Link
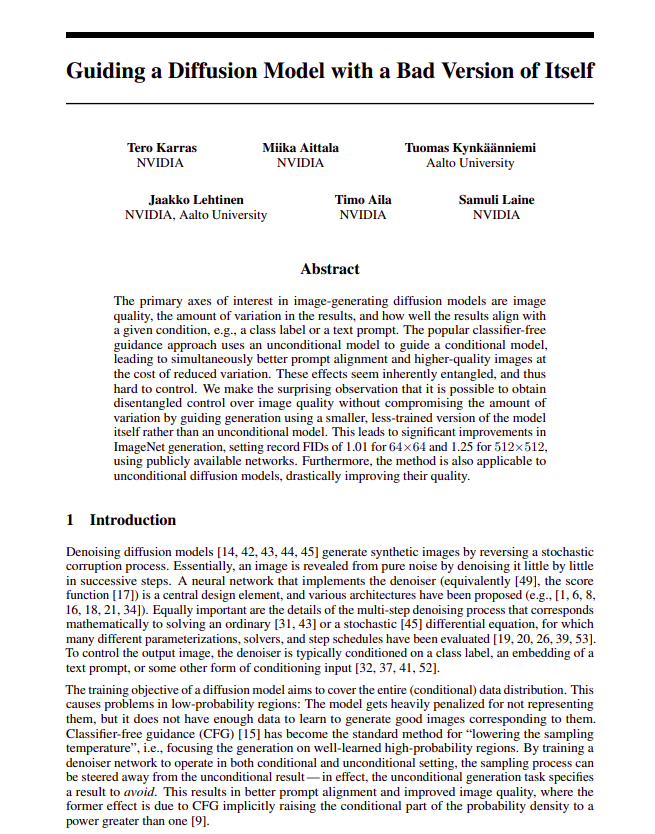
Author: Tero Karras, Miika Aittala, Tuomas Kynkäänniemi, Jaakko Lehtinen, Timo Aila, Samuli Laine
Challenging the conventional Classifier-Free Guidance (CFG) used in text-to-image (T2I) diffusion models, this paper introduces Autoguidance. Instead of relying on an unconditional term (as in CFG), Autoguidance employs a less-trained, noisier version of the same diffusion model. This approach improves both image diversity and quality by addressing limitations in CFG, such as reduced generative diversity. The paper’s innovative strategy offers a fresh perspective on enhancing prompt alignment and T2I model outputs.
NeurIPS 2024 Best Paper in the Datasets & Benchmarks Track
Here are the best papers in the Datasets & Benchmarks Track
The PRISM Alignment Dataset: What Participatory, Representative, and Individualized Human Feedback Reveals About the Subjective and Multicultural Alignment of Large Language Models
Here’s the Paper: Link
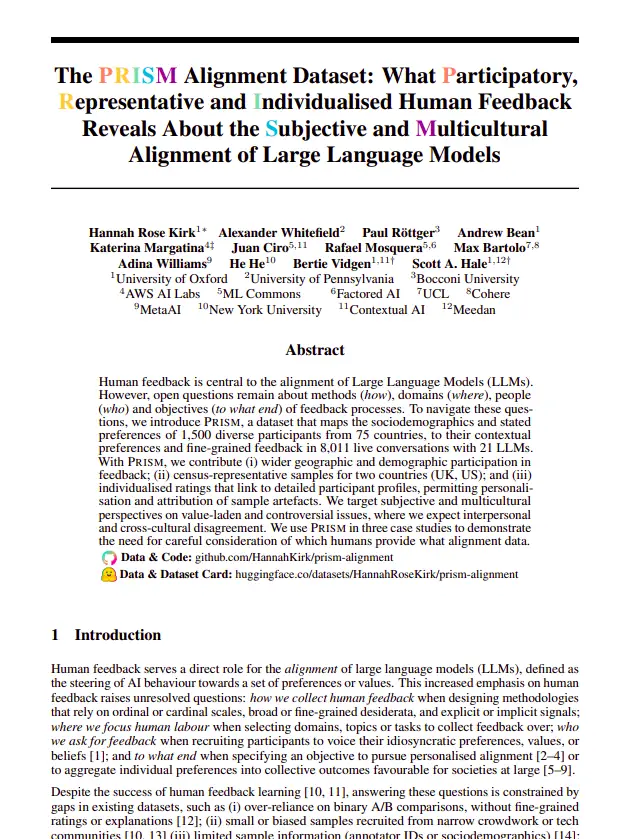
Author: Hannah Rose Kirk, Alexander Whitefield, Paul Röttger, Andrew Michael Bean, Katerina Margatina, Rafael Mosquera, Juan Manuel Ciro, Max Bartolo, Adina Williams, He He, Bertie Vidgen, Scott A. Hale
The PRISM dataset stands out for its focus on the alignment of LLMs with diverse human feedback. Collected from 75 countries with varying demographics, this dataset highlights subjective and multicultural perspectives. The authors benchmarked over 20 state-of-the-art models, revealing insights into pluralism and disagreements in reinforcement learning with human feedback (RLHF). This paper is especially impactful for its societal value, enabling research on aligning AI systems with global and diverse human values.
Committees Behind the Excellence
The Best Paper Award committees were led by respected experts who ensured a fair and thorough evaluation:
- Main Track Committee: Marco Cuturi (Lead), Zeynep Akata, Kim Branson, Shakir Mohamed, Remi Munos, Jie Tang, Richard Zemel, Luke Zettlemoyer.
- Datasets and Benchmarks Track Committee: Yulia Gel, Ludwig Schmidt, Elena Simperl, Joaquin Vanschoren, Xing Xie.
Here are last year’s papers: 11 Outstanding Papers Presented at NeurIPS
Open-source AI: Year in review 2024
Here’s the Space: huggingface/open-source-ai-year-in-review-2024
Just scroll in the given window and click on “The NeurIPS Class of 2024”
1. Top Contributors Globally
- Massachusetts Institute of Technology (MIT) leads with the highest contribution at 3.58%.
- Other top institutions include:
- Stanford University: 2.96%
- Microsoft: 2.96%
- Harvard University: 2.84%
- Meta: 2.47%
- Tsinghua University (China): 2.71%
- National University of Singapore (NUS): 2.71%
2. Regional Insights
North America (Purple)
- U.S. institutions dominate AI research contributions. Major contributors include:
- MIT (3.58%)
- Stanford University (2.96%)
- Harvard University (2.84%)
- Carnegie Mellon University (2.34%)
- Notable tech companies in the U.S., such as Microsoft (2.96%), Google (2.59%), Meta (2.47%), and Nvidia (0.86%), play a major role.
- Universities such as UC Berkeley (2.22%) and the University of Washington (1.48%) also rank high.
Asia-Pacific (Yellow)
- China leads AI research in Asia, with strong contributions from:
- Tsinghua University: 2.71%
- Peking University: 2.22%
- Shanghai Jiaotong University: 2.22%
- Chinese Academy of Sciences: 1.97%
- Shanghai AI Laboratory: 1.48%
- Institutions in Singapore are also prominent:
- National University of Singapore (NUS): 2.71%
- Other contributors include Zhejiang University (1.85%) and Hong Kong-based institutions.
Europe (Red)
- European research is robust but more fragmented:
- Google DeepMind leads in Europe with 1.85%.
- ETH Zurich and Inria both contribute 1.11%.
- University of Cambridge, Oxford, and other German institutions contribute 1.11% each.
- Institutions like CNRS (0.62%) and Max Planck Institute (0.49%) remain important contributors.
Rest of the World (Green)
- Contributions from Canada are noteworthy:
- University of Montreal: 1.23%
- McGill University: 0.86%
- University of Toronto: 1.11%
- Emerging contributors include:
- Korea Advanced Institute of Science and Technology (KAIST): 0.86%
- Mohamed bin Zayed University of AI: 0.62%
3. Key Patterns and Trends
- U.S. and China Dominate: Institutions from the United States and China lead global AI research, accounting for the majority of contributions.
- Tech Companies’ Role: Companies like Microsoft, Google, Meta, Nvidia, and Google DeepMind are significant contributors, highlighting the role of industry in AI advancements.
- Asia-Pacific Rise: China and Singapore are steadily increasing their contributions, demonstrating a strong focus on AI research in Asia.
- European Fragmentation: While Europe has many contributors, their individual percentages are smaller compared to U.S. or Chinese institutions.
The NeurIPS 2024 contributions underscore the dominance of U.S.-based institutions and tech companies, coupled with China’s rise in academia and industry research. Europe and Canada remain critical players, with growing momentum in Asia-Pacific regions like Singapore.
Conclusion
The NeurIPS 2024 Best Paper Awards celebrate research that pushes the boundaries of machine learning. From improving the efficiency of LLMs to pioneering new approaches in image generation and dataset alignment, these papers reflect the conference’s commitment to advancing AI. These works not only showcase innovation but also address critical challenges, setting the stage for the future of machine learning and its applications.
By Analytics Vidhya, December 16, 2024.